|
 |
|
Computer Logical Design Laboratory |
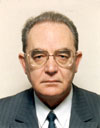 Vyacheslav. B. Marakhovsky Professor |
In 2002, the laboratory research has been conducting in three directions: On-Chip Learning of neuron-MOS Artificial Neuron, CMOS beta-Driven Threshold Elements and Arti??cial Neurons, and CMOS Neuro-Fuzzy Circuits and Devices.
In the first direction, we continued to solve the problem of on-chip learning for the artificial neuron based on floating gate transistors. Design methodology of a learning structure was created. This methodology paid respect to transistor parameters and dimension deviations. Especially important problem is providing tolerance of the neuron to supply voltage deviations. As a result of the investigation, the main parts of the structure have been developed. The proposed structure was fully tolerant to any kind of deviations and used the idea of parameter deviation compensation. It was shown that such artificial neuron could be successfully learned to realize threshold functions of not more then 7 variables. Digital-analog CMOS threshold elements and artificial neurons have been very popular for some application during the last decade. One of the important characteristics of these devices is the degree of their implementability, i.e. the restriction on the class of the threshold functions that can be reliably implemented by a single element under the drift of technological and environmental parameters. For most available implementations, this restriction is defined by the biggest permissible sum of the inputweights and threshold. Previouslywe have suggested new CMOSbeta-driven threshold element, for which the limiting parameter is only the threshold value. The idea of such threshold element is based on the representation of the threshold function in the ratio form. It allows implementing threshold functions as a ratio of conductivities of n- and p- chains of CMOS device. In the second direction, we studied the problems of increasing functional power of an artificial neuron on the base of the beta-driven CMOS threshold element. The following results were
- The beta-driven artificial neuron learnable to non-isotonous threshold functions was studied. We suggested the neuron synapse capable to form the weight and type (excitatory or inhibitory) of the input during the learning using only increment and decrement signals. The neuron with such synapses can be learned to an arbitrary threshold function of a certain number of variables.
- Synapse circuits were suggested with two memory elements for storing positive and negative inputweights along with the procedure of on-chip learning. The results of SPICE simulation proved that the problem of neuron teaching to non-isotonous threshold functions has been successfully solved.
Third direction is connected with the problem of designing CMOS Neuro-Fuzzy Circuits and Devices. During several last decades for solutions of sophisticated control problems and data processing effectively developed neuromorphicmethods, i.e. methods inspired with knowledge of processes in a nervous system. The special place among these methods takes ANN (Artificial Neural Networks). ANN can be realized as software implementation on universal or specialized processors. Alternative to this is analog-digital hardware implementation of the ANN. The main advantage analog-digital implementation as contrasted to software implementation is the principled increase of relation throughput/complexity. Themain lack of this implementation is the limitation on implementability, i.e. on complexity of functions implemented by one element. Increasing of above relation is the main result of the beta-driven circuitry application. The ecological niche for analog-digital ANN actuates: image preprocessing (artificial retina etc.), intellectual fuzzy controllers, robotic control (locomotion, scrub moving etc.), pattern recognition, fault detection, and many others. The research in this direction implies the creation of methods and tools of designing full and semi-custom neuro-fuzzy VLSI and embedded devices and systems on the base of this circuitry. These methods and tools include creating threshold elements and devices, learnable beta-driven artificial neurons, fuzzy threshold elements and devices; embedded neuro-processors, neuro-arrays and fuzzy controllers and correspondent IP (intellectual properties); design methods and design know-how for devices and systems on the base of beta-driven circuitry.
|
[marak-01:2002] | V. Varshavsky and V. Marakhovsky. GALA (Globally Asynchronous - Locally Arbitrary) Design. Lecture Notes in Computer Science 2549, Concurrency and Hardware Design, Advances in Petri Nets, LNCS 2549:61-107, 2002. The problem of organizing thet emporal behavior of artificial systems is discussed. This problem is mainly associated with providing the interface between physical (natural) and logical (artificial) time. The most widely spread method is using a system clockthat allows to remove physical time from the behavior models. There is a number of algorithms easily formulated in logical time for which we encounter great difficulties trying to formulate them for the asynchronous case. The suggested GALA (Globally Asynchronous - Locally Arbitrary) design methodology is based on decomposing the system to Processors Stratum and Synhro-Stratum. The synchro-stratum acts as a distributed asynchronous clock that produces local synchro-signals for the processor stratum, which basically is the synchronous prototype. A synchro-stratum, like any asynchronous circuit, interacts with the external devices (processor stratum) on the base of handshake. Every local device produces the acknowledgment signal and send it to synchro-stratum. The designer can use the whole range of methods to implement this signal (Locally Arbitrary): from self-timed design to incorporated parallel delay. For various disciplines of prototype synchronization, the corresponding synchro-stratum implementations are suggested. GALA methodology is illustrated on examples of device design, such as counter with constant response time, one-two-one track FiFo, arbitration-free counterflow processor architecture. |
Referred Proceeding Papers |
[marak-02:2002] | V.and Levin I. Varshavsky, V.and Marakhovsky. CMOS Based Beta-Driven Threshold Elements with Functional Inputs. In Proceedings of thee 22-th IEEE Convention of Electrical and Electronics Engineering in Israel, pages 111-113, Tel-Aviv, Israel, Dec. 2002. IEEE, IEEE Press. This paper is focused on a functional capability extension of threshold elements. Functional inputs were introduced into the beta-driven threshold element. It was proved that such element with functional inputs can realize arbitrary monotonic Boolean function. The threshold element CMOS implementation having the proposed functional inputs was suggested. SPICE simulation results demonstrated the high efficiency of the solutions. |
[marak-03:2002] | V. Marakhovsky, 2002. Member of IEEE |
[marak-04:2002] | V. Marakhovsky, 2002. Member of ASM |
[marak-05:2002] | Kouji Watanabe. Graduation Thesis: A Neuron-MOS Threshold Element with Switching Capacitors, University of Aizu, 2003. Thesis Advisor: Marakhovsky, V. |
[marak-06:2002] | Takanobu Asano. Master Thesis: Arti??cial Neuron CMOS Implementation Learnable to an Arbitrary Threshold Function, University of Aizu, 2003. Thesis Advisor: Marakhovsky, V. |
[marak-07:2002] | Takaaki Asakura. Master Thesis: Design Methodology for CMOS based Beta-Driven Threshold Elements, University of Aizu, 2003. Thesis Advisor: Marakhovsky, V. |
[marak-08:2002] | Yutaka Nemoto. Master Thesis: Learning Circuit Implementation with Sourse Voltage Deviation Tolerance Structure for the neu-MOS Artificial Neuron, University of Aizu, 2003. Thesis Advisor: Marakhovsky, V. |
[marak-09:2002] | Hirotoshi Takagi. Master Thesis: Deviation-Tolerant Weight Voltage Refresh Circuit for On-chip Neuroal Networks, University of Aizu, 2003. Thesis Advisor: Marakhovsky, V. |
[marak-10:2002] | Up to the 1st of August, 2002: Chair of the Computer Systems Department of the Graduate School. |
|