Adaptive Real-time
Anthropomorphic Robot Hand
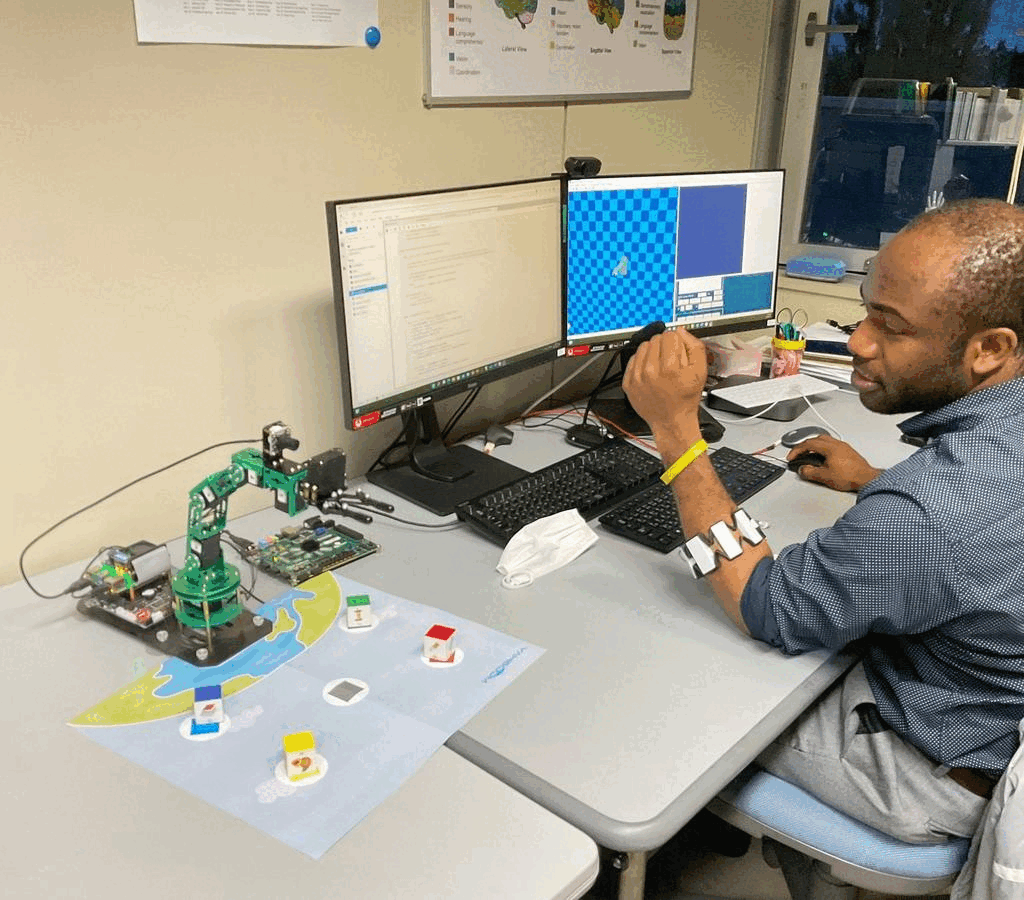
Prosthetic limbs can
significantly improve the quality of life
of people with amputations or neurological
disabilities. With the rapid evolution of
sensors and mechatronic technology, these
devices are becoming widespread
therapeutic solutions. However, unlike
living agents that combine different
sensory inputs to perform a complex task
accurately, most prosthetic limbs use
uni-sensory input, which affects their
accuracy and usability. Moreover, the
methods used to control current prosthetic
limbs (i.e., arms and legs) generally rely
on sequential control with limited natural
motion and long training procedures.
We are developing an advanced myoelectric
real-time neuromorphic prosthesis hand,
AIzuHand, with sensory integration and
tactile feedback. In addition, we
investigate a user-friendly software tool
for calibration, real-time feedback, and
functional tasks.
|
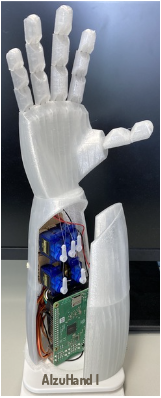 |
Features:
- Name: AIzuHand I
- Total Weight: 422g (276g
without controller)
- Control: sEMG
- DoF: 5
- Feedback: No
- Mode: AN/SN
|
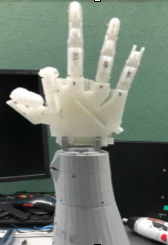 |
Features:
- Name: NeuroSys
- Weight: 492g
- Control: sEMG, RM
- DoF: 7
- Feedback: No
- Mode: AN/SN
|
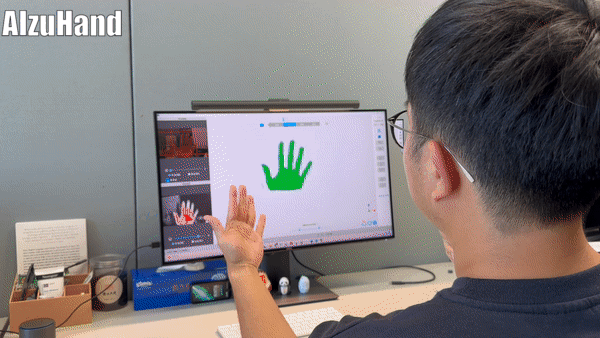
|
|
- Cheng Hong, Sinchhean Phea,
Khanh N. Dang, Abderazek Ben Abdallah,
''The AIzuHand Neuromorphic Prosthetic
Hand,'' ETLTC2023, January 24-27, 2023.
A myoelectric prosthetic allows
manipulation of hand movements through surface
electromyogram (EMG) signal generated during
muscle contraction. However, the lack of an
intuitive personal interaction interface leads
to unreliable manipulation performance. For
better manipulation, sEMG-based prosthetic
systems require the user to understand their
EMG signal levels and adjust the thresholds
for better movements. A practical prosthetic
is still being challenged by various factors
inherent to the user, such as variation in
muscle contraction forces, limb positional
variations, and sensor (electrode) placements.
Thus, a personal interface that can adjust the
prosthetic parameters across amputees is
required. In addition, this interface is also
needed for some targeted users in pediatrics
who require external assistance for control.
In this work, we present a low-cost real-time
neuromorphic prosthesis hand, AIzuHanda, with
sensory motor integration. We aim to develop
solutions for controlling prosthetic limbs to
restore movement to people with neurologic
impairment and amputation. The AIzuHand system
is empowered by a user-friendly mobile
interface (UFI) for calibration, real-time
feedback, and other functional tasks. The
interface has visualization modules, such as
an EMG signal map (EMG-SM) and mode selection
(MS), for adjusting the parameters of the
prosthetic hand. Furthermore, the interface
helps the user establish a simple interaction
with the prosthetic hand.
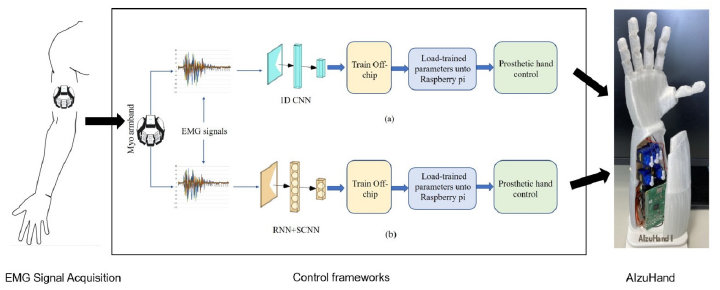
|
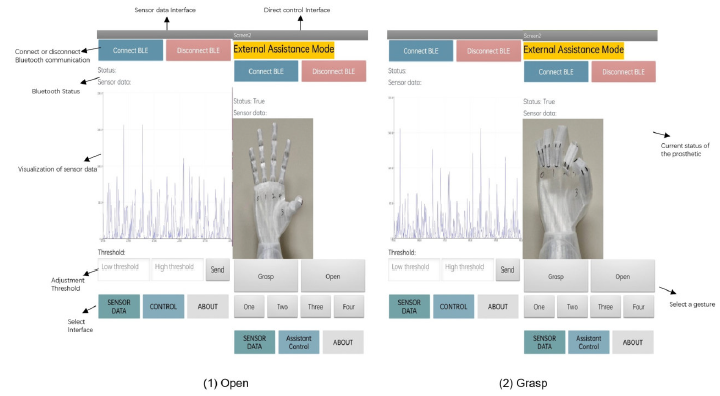 |
|
- Mark Ogbodo, Abderazek Ben
Abdallah, ''Study of a Multi-modal
Neurorobotic Prosthetic Arm Control
System based on Recurrent Spiking Neural
Network,'' ETLTC2022, January 25-28,
2022
The use of robotic arms in
various fields of human endeavor has increased
over the years, and with recent advancements
in artificial intelligence enabled by deep
learning, they are increasingly being employed
in medical applications like assistive robots
for paralyzed patients with neurological
disorders, welfare robots for the elderly, and
prosthesis for amputees. However, robot arms
tailored towards such applications are
resource-constrained. As a result, deep
learning with conventional artificial neural
network (ANN) which is often run on GPU with
high computational complexity and high power
consumption cannot be handled by them.
Neuromorphic processors, on the other hand,
leverage spiking neural network (SNN) which
has been shown to be less computationally
complex and consume less power, making them
suitable for such applications. Also, most
robot arms unlike living agents that combine
different sensory data to accurately perform a
complex task, use uni-modal data which affects
their accuracy. Conversely, multi-modal
sensory data has been demonstrated to reach
high accuracy and can be employed to achieve
high accuracy in such robot arms. This paper
presents the study of a multi-modal
neurorobotic prosthetic arm control system
based on recurrent spiking neural network. The
robot arm control system uses multi-modal
sensory data from visual (camera) and
electromyography sensors, together with
spike-based data processing on our previously
proposed R-NASH neuromorphic processor to
achieve robust accurate control of a robot arm
with low power. The evaluation result using
both uni-modal and multi-modal input data show
that the multi-modal input achieves a more
robust performance at 87%, compared to the
uni-modal.
-
Abderazek Ben Abdallah ,
Zhishang Wang, K. N. Dang, Masayuki
Hisada, '' Lacquering
Robot System [漆塗りロボットシ
ステム],' 特願2024-056380 (Macrh 29,
2023)
The
'Self-Controlled Urushi Robot Hand
Painting System' is a cutting-edge robotic
system designed to meet the evolving needs
of modern Urushi painting. It skillfully
manages a wide range of customer orders,
interprets request formats such as images,
text, or speech, and effectively handles
the Urushi painting process. The system
also ensures efficient task allocation
based on robot hand 's availability and
evaluates the adequacy of materials. It
includes a blockchain platform that
enables secure Urushi trading by
generating digital certificates for the
authenticity, preserving the integrity of
traditional Urushi craftsmanship in a
modern technological environment. In
addition, robot hand intelligently
monitors material inventory and
autonomously procures the needed material
by either using its profits or debiting
the necessary amounts from linked bank
accounts.
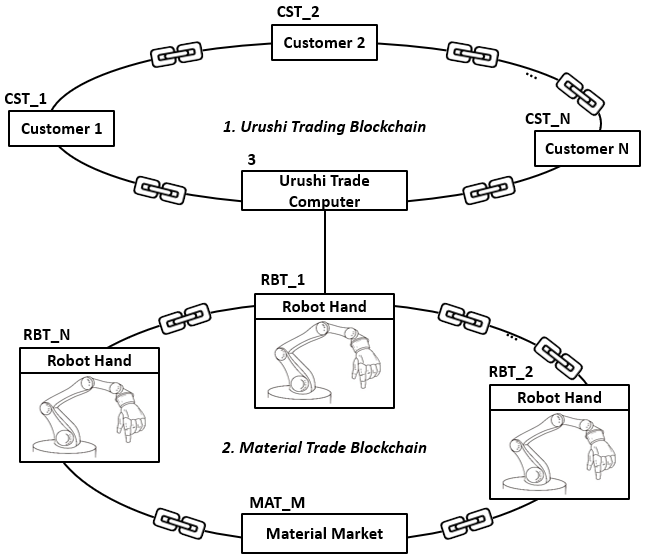
|
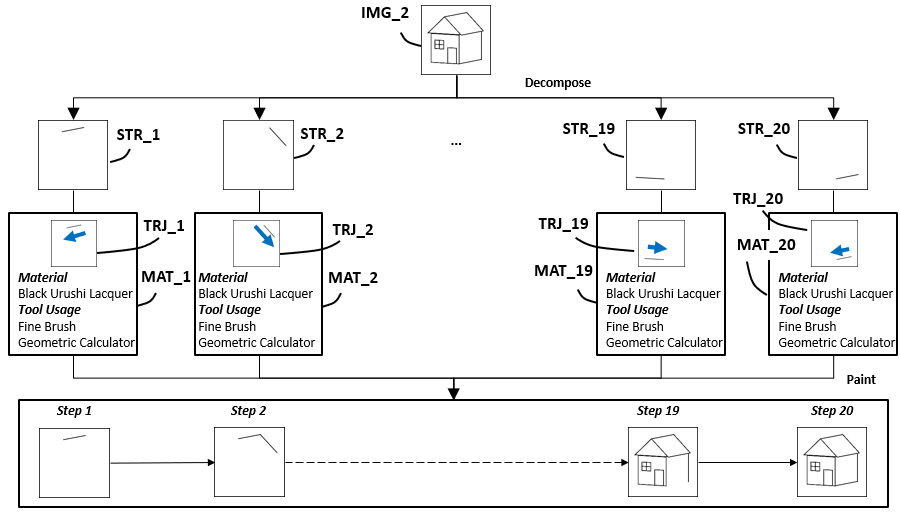
|
|
|